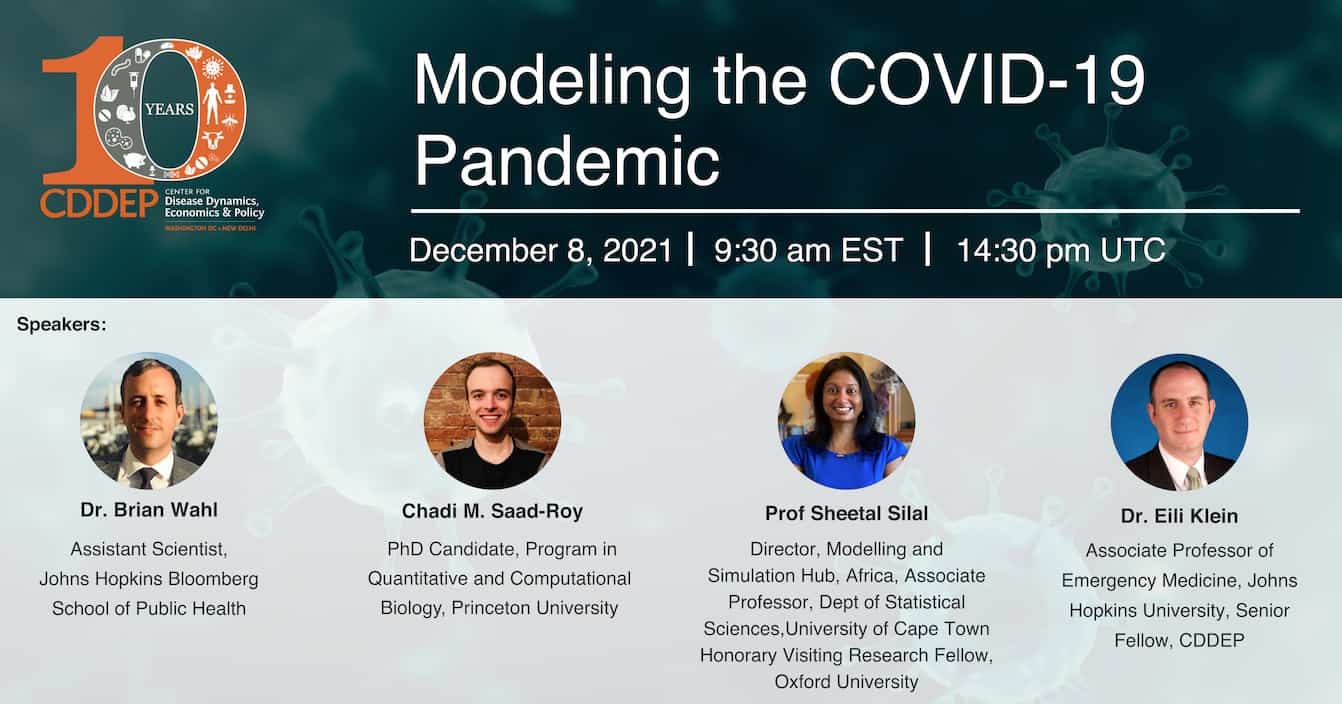
You can also view a recording of this webinar here.
Erta Kalanxhi
Hello everyone, and thank you for joining us. Welcome to CDDEP’s conversation series on global health. This is our ninth webinar.
Today we’ll discuss the utility of mathematical modeling and understanding disease dynamics and informing policy in the context of the COVID-19 pandemic. I’d like to thank our panel today for joining us to share their views and experiences because we all know that this is a very busy time, especially for modelers these days.
We’re very happy to have with us Dr. Eili Klein, a senior fellow at CDDEP and associate professor of Emergency Medicine at Johns Hopkins. We have Dr. Sheetal Silal from the University of Cape Town Dr. Silal is the Director of Modelling and Simulation Hub Africa and Associate Professor at the Department of Statistical Sciences at the University of Cape Town. We also have Dr. Brian Wahl, assistant scientist at the John Hopkins Bloomberg School of Public Health. We also have Chadi Saad-Roy, Ph.D. candidate at the Quantitative and Computational Biology department at Princeton University.
We will start the webinar with some specific questions for the speakers but we will have about 10-15 minutes at the end of the hour to answer your questions. We see that we have a very international audience today, so we look very much forward to the questions you might have. You can include them in the q&a section in the Zoom app. Before we start today, Dr. Ramanan Laxminarayan, director at CDDEP, would like to say a few words. So over to you, Ramanan.
Ramanan Laxminarayan
Thanks, Erta. And welcome to all of you. As Erta mentioned, this is the ninth in our series. It’s been a really exciting series, we’re going to hope to close with 10, so there’s only one left.
Modelling has been something that’s very core at CDDEP. It is also something which is taking up a lot of our time, particularly the COVID modelling, since last February, when we knew that we had something to really worry about here.
We’ve done this not just in the United States, which is what you’ll hear from Eili, for instance, in the state of Maryland. You’ll hear about, the work that has been done in South Africa from Dr. Sheetal Silal and the excellent work that Chadi has done with a group at the Department of Ecology and Evolutionary Biology at Princeton.
Last but not least, Brian Wahl’s got some amazing work on disease modelling with respect to COVID and especially COVID epidemiology that’s about to come out, which is based on data from India and the state of Uttar Pradesh where he works.
The challenge of modelling COVID has simply been that the questions asked of models have often run far ahead of the data or even knowledge about the virus. But at the same time, I think it has been a great opportunity to educate people outside of, or perhaps even within, health on the role that mathematical models can play.
I think that knowing what models can do, but also knowing what they can’t do, is really, really important, and I think we need to see them as guideposts rather than exact predictors of what will actually happen.
At CDDEP itself, we could at the very start of the pandemic “predict” that something as fast moving as COVID would end up resulting in hundreds of millions of cases in India, for instance. But sometimes the modelling also needs to be accompanied by a communication exercise where people are able to understand what this really means.
We’re saying things in fairly neutral terms: out of the hundreds of millions of cases, the vast majority might be mild. But people are scared of a new virus and of infections, and, therefore, the response might not be quite what we want. It turns into fear or the converse, which is that you say that this is not a lethal variant, for instance. If someone were to say that, then the response tends to be much more of taking the variant very likely and not really protecting themselves.
Last but not least, every time I’m asked this question on any sort of media about the modelling, I often say two things: One is that anything you say today will probably be out of touch in two weeks because the science would have changed, or our information set would have changed. The second is that of the three factors that determine how diseases move – the virus, human behavior and government policy – the most predictable is the virus, the less predictable is human behavior, and the least predictable is government policy.
I think we’ve seen this in all waves of this pandemic, particularly during Omicron. The most recent revelation that we have a new variant, which again, was no surprise that we would have one, but of course the responses have been absolutely astounding – and not in a good way. This really reflects poor understanding of what the science is trying to communicate and what people, both the public and the policymakers, are hearing.
The challenge of modelers is no longer of building great models. It’s really of building models that can anticipate some of these, in terms of behavior and government policy. But then the challenge for models is also in terms of communicating to a much wider audience than we’ve ever had to do before.
We’re delighted to have four of the best people working on this around the world and I look forward to hearing what they have to say. Thank you Erta.
Erta Kalanxhi
Thank you, Ramanan. We are ready to start with Chadi from Princeton University. Starting from the earliest infectious disease models, epidemiologists have tried to use models to explain and predict disease dynamics. In your opinion, how does modelling serve in understanding disease transmission? And how did this change with the COVID-19 pandemic, especially following the increase in data availability?
Chadi Saad-Roy
That’s a great question. Thank you very much for having me, it’s my pleasure to be here and to participate in this conversation.
To really address this question properly, we have to go back to a long time ago. This field has a rich history and foundations probably start with Daniel Bernoulli in 1766, looking at pandemic smallpox.
These concepts are pretty historical and then, more recently, have been formalized with things that we know better such as the SIR model, where individuals are susceptible and affected and then recovered. This was introduced in 1927 by Kermack and Mckendrick, and then Ronald Ross, big pioneer of mathematical modelling as well, on malaria. Since then, it’s been refinements and a number of developments in mathematical, statistical, and biological literatures.
I think it’s been a long time since a lot of these issues have been present, and a lot of theory has been developed. But the COVID-19 pandemic has really brought these to light and public discourse, really. I was trying to make a list of the things that models can be useful for and obviously my list is very, very limited, and probably there are many more uses.
You can use models to determine exponential growth rates: how fast are cases growing? That ties into determining the basic reproduction number- the number of new infections that arise from a single infection in a fully susceptible population. Related to this is the dispersion of infectiousness. These models can help us infer that and then we can learn something about the biology of the disease, which then tells us something about the public health interventions we need to place.
On a different side of things, you can also use models to aid in underestimating reporting of cases. Not every infection leads to positive test. A lot of individuals just won’t get tested, and that’s an under reporting.
This is a severe issue, and prediction and models can aid us to determine this underreporting rate. There is also trying to use models to predict or forecast much more, finally, what will happen and so a lot of there’s been huge push for doing that. And I think that’s what the public wants – what’s going to happen in the next few weeks? That’s usually a very arduous task, but an important one, nonetheless. A number of very great groups have done substantial leaps in this front.
And then there’s the work I’ve been more involved in, which is more to generate possible qualitative outcomes and see how they’re shaped by different uncertainties. There are many more uses, but if we focus in on my own work, we can see as an illustrative example of how modelling can progress throughout pandemic.
We were initially looking at initial uncertainties with respect to the strength and duration of immunity and how if you properly account for this in models, then you can get a really wide range of outcomes. Back then, vaccines were in development, but we didn’t really know what shape or form they would take.
Then vaccines were developed and the question became, should you space the two doses and deviate from the manufacturer’s recommended spacing? or should you not? There’s a lot of epidemiological considerations and evolutionary ones.
The next thing we did was refine this model and we were able to evaluate the possible outcomes by general qualitative predictions: what might happen depending upon what the parameters are. More recently, which is still at the forefront of everyone’s mind now again, is this question of vaccine equity and driven by vaccine nationalism. We examined extensions of our previous work: what happens if certain countries have high access and others have low access?
In my own work, you can see the trajectory as the questions arise in the public health discourse that we’re addressing. This is an open-ended question, but hopefully, I’ve addressed some of the key issues.
Erta Kalanxhi
Yes, thank you very much. Thank you, Chadi, for that introduction. We have one more question for you. It has to do with the conceptual limitations of modelling. Models provide a framework to understand complex concepts and what are the current conceptual limitations with modelling studies?
Chadi Saad-Roy
That’s another great question and actually ties into the end of my answer to the first question. As I was describing, so we’ve had the simple model that accounts for the strength and duration immunity, and once we refined that to two doses, then the model increased dramatically in complexity.
Usually you can make very complex simulations, complex models, that are with tremendous complexity and that can replicate and encompass a lot of important aspects, but they’re much less tractable. On the other hand, you can make simple models that are tractable, but then they gloss over a number of important features, and they make assumptions about a number of important features.
I think the idea would be to try to increase tractability of more complicated models to properly account for different behavior. I think is a major limitation.
As a specific example, beyond my own work, we can think of the interplay of human behavior and disease dynamics. This is a tremendous problem. There’s been huge development in this area, but the models that you use to generate this will be along this range: the simpler you go, the more you’re glossing over and probably the less accurate predictions might become.
You really have to balance this always and figure out how complicated you want the model to be. Even when we extended to vaccine nationalism, we only have two countries, so the simplest possible extension already exists. For most of the details, you have to rely upon numerical simulation and the tractability there is lost.
However, on the other hand, I think the pandemic has led to a number of interesting breakthroughs on previous conceptual limitations. There’s some interesting work by James Haydel 2021 in Science, where they use Ct values to infer epidemiological dynamics.
They are using this abundance of data to really try to predict shape of the pandemics. There’s also been this great collaboration between engineers, biologists and interdisciplinary scientists to understand transmission and aerosols and droplets and how these things function with humidity and temperature and environment.
I think a good citation for this might be borecell eli 2021(14:29) You’re using this novel data, which usually is what conceptually limits the models: there’s this limitation of data that feeds back the limitation of the model. Individuals have been able to propel the field forward by combining new types of data with novel modelling approaches, which probably will lead to a number of works in math, stats, and biology, and with public health relevance. Hopefully that addresses your question Erta.
Erta Kalanxhi
Yes. Absolutely, thank you so much. I was just going to add that if you’d like to share a link to the papers you’re referring to or any relevant recent articles from your work, please feel free to share it in the chat. Thank you very much.
Then, Eili, we have a few questions for you on the complexity of mathematical modeling. How can more complex models help to understand the multiscale effects of diseases? For example, is it possible to integrate models to understand how within those dynamics of diseases can impact population level disease transmission?
Eili Klein
With regardless to the first question on complexity, I think the big question is what is the goal of the model? Whenever I talk about modelling, it’s almost impossible not to show the quote by George Box: “all models are wrong, but some are useful.”
Simple models are unlikely to represent any system exactly, but parsimonious models can often provide remarkably useful approximations. As the complexity of a model increases – and in recent years the ability to meet more and more complex models has increased dramatically – the question still remains: what’s to be gained by adding all that complexity?
As Chadi had said, the greater the complexity of that model, the harder it is to evaluate and understand what’s going on. If we go all the way back to the chemistry where a lot of these infectious models came from, the ideal gas law, right, PV equals RT. The ideal gas law relates pressure, volume and temperature to describe how gases will behave in ideal conditions. Is this model true? I don’t think that’s actually the right question.
The right question is whether this model is useful and illuminate because understanding deviations from this model allow you to understand important aspects of chemistry. I bring up chemistry because the SIR model, in general, comes from chemistry. It’s a concept, the same way that atoms move around independently and interact and convert from one state to another. That’s how models of infectious disease were originally built.
We can make those more complex; we can add different aspects to that. The question is specifically within host dynamics: do within host dynamics impact population level disease transmission? The question I would ask more, in a different way, is how do the dynamics at the individual level actually scale up and matter?
You can attack this from sort of a bottom-up approach or a top-down approach. From a bottom-up perspective, the question is how do all of these interactions at the individual level actually matter? You can build a model of how within host dynamics actually scale, whether is COVID or some other disease, and then scale up those and connect those individuals, and see how that affects population.
On the other hand, you could also start from a model that is simpler, where everyone is basically the same, and start adding variation individuals to see where and what level of variation actually matters or is biologically relevant. That is more of a top-down approach. Those are two ways to get at this question of how do multiscale dynamics actually matter and when should they be put together into models to sort of define how you want to move forward?
I think there’s a lot of different ways you can answer the question of complexity. The questions really come down to what is the question you are trying to answer. I’ll talk a little more about this in the second question, but I think the issue really is, what are we trying to answer? Do you want to know the specifics of how multi scales actually impact the dynamics? Do you really want to understand the model at the individual level?
They actually just built a model recently for how the dynamics of the scale of a single Byron inside a droplet actually work, and they ran that model for a total of exactly 1,000,000th of a second. They had to use the biggest computers that are available now and the amount of data that was generated from that will take years to even analyze.
You can’t build that into another model, but you can take the dynamics and the importance of that and understand which of these parts of the model matter. Is it the network part of model, how populations are connected that really matters? Is it the airflow and the droplets that really matter? Or is it within host dynamics within an individual host that matter?
For every disease it’s going to be different, so how you want to design those models is going to be different. Realistically, no one model should include all of those things because it’s going to be too complex. The question is how do you put together these arguably simpler models- that hopefully have some parsimony to them or are tractable that can provide answers that can you can use- to define either specifics about the disease that are important, or specifics about how you want to deal with public policy.
Both of those things actually have different frameworks in terms of how you want to deal with the outcomes. The last thing I mention is on same lines. If I am asked to answer questions about public policy, I’m going to build a different model than if I’m trying to answer questions about where we want to build science.
I might want to build a within host model to sort of define the questions that might be useful to ask in terms of what experiments might be necessary, rather than how I’m going to build that individual within host model to answer questions on public policy level. Those two things are different modelling techniques and have different frameworks and outcomes.
Erta Kalanxhi
Thank you, Eili, that’s fascinating stuff. You have already touched upon my second question, but I will ask it anyway, in case you want to revisit parts of it. As more data becomes more readily available, is it beneficial to continue refining models with moderate complexity? And what will the known and unknowns be once we have enough data to model behavior and diseases correctly?
Eili Klein
I think again, this gets back to the question that one is trying to answer. The problem is that a lot of what people view modelling for and what people talk about modelling in the context of COVID is how are we going to do prediction and forecasting.
As I was sort of saying, there are there are lots of models that can provide utility. So that within hosts model may not be need to be coupled to population level model to provide utility. There is a lot of different factors that you can look for. When you start to talk about big data, the really crucial question, that we need to start looking for and understanding in terms of modelling, is what data is needed.
Chadi mentioned that somebody used Ct values to help try to understand the dynamics of disease. In 2010, I saw a talk where someone was using a home-built app to track some phones. People signed up and they were showing how individual tracks people moved and how their regular movement patterns defined important characteristic of daily interactions.
I remember discussing how great it would be if we could have that at a population level, because then we could track how diseases spread. Well, here we are in 2021, and we actually have data at somewhat of this scale.
Particularly in the US, there has been all of these mobile phone data providers that actually provided this data for free. We were all excited we started using that model.
If you at the looked at the original data that people started using back in March 2020, there was tremendous correlation between drops and movement based on the mobile phone data and transmission. Everyone thought we can use this data to provide important information, but then everyone started moving around again and there was no correlation with transmission.
The question came of what is the mobile phone data telling us? What are the important characteristics of it? We started to dive into that data and find there’s all sorts of biases: Who has the who actually has a mobile phone? Who’s actually involved in transmission? If two people are near each other with mobile phones, does that mean they’re actually transmitting?
There’s a lot of other factors that we presumably are missing that are not provided within that data. Some people are trying to just throw lots of big data at a model and try to figure things out using AI. But at a general level, I think the bigger questions are really what are the types of data that we need to collect? And what are the types of data that we need to use to inform important aspects of the model?
That gets to this question of what does everybody really want to do with models? I think a large portion of it is that people want to do forecasting. There’s a been a huge push within the US for this specifically. Congress actually appropriated money to generate a new pandemic forecasting center.
The idea is the way we developed weather models. It’s useful to think about how accurate weather forecasting is, and I don’t think people have fully understood the weather parable. I’m not going to argue that weather forecasts haven’t dramatically improved: weather forecasting has become really, really reliable, in some instance.
It was driven by the accumulation of lots and lots of big data. First, we had radar, which emerged in the 40s, and that helped provide some predictability. Then we have weather satellites, ground level humidity collections, and all of these different data streams which all are all pushed into these models that can provide two types of models.
The first is short term weather forecasting. We can see this in this slide here, where you can see hurricane forecasting. Hurricane forecasts have actually become really good, but there are actually lots of agglomerations of models. Back in 2012, with Hurricane Sandy, you have all these different models, and many of them have predicted this track where it went to the left.
This is what you see typically on TV where you get this cone of uncertainty, which comes from all of these different models. You’ll see even one of two of the models have just very, very different outcomes. There’s this concept that they’re sort of put together – putting lots of models together.
Those forecasts were pretty accurate at about two or three days, which is useful. Now I have an app on my phone which tells me what the weather is going to be like in the next 15 minutes; also, very useful for what I’m doing in the next couple hours. Unlike weather, disease is very different.
If I make a forecast for the weather, whether or not people change behavior if it’s going to rain isn’t going to change the fact that it’s going to rain. On the other hand, if you make a forecast for disease, that can actually change how people are going to behave, which ends up changing the outcome. The important question, though, is what are people going to do with the with these forecasts?
I think that’s where the difference and the weather parallel differs. Realistically, many of these mechanistic models and all of these modelling systems that have been put together by CDC have been pretty good at predicting stuff about two weeks out.
The question is, what is two weeks out? Is that useful in a disease context, or do we need something different? Even if they’re accurate, what are we going to do about it? All of these weather predictions, all of these things have told us that we can now go and do specific things to try to save lives. We have hurricane warnings and we evacuate certain areas.
What are we going to do if the forecast for flu is high? Will that change how people go about their day? Do we want to have people wear masks? In certain contexts, do we mandate that or do we just suggest it? Maybe we want to change how schools are in concession and as we can see, at least in the US, that has caused significant complications. Trying to adjust and force how people behave becomes problematic in many ways.
We can get lots and lots of big data, and we can maybe improve our models, but I think Ramanan alluded to this right at the beginning, those model predictions have to drive something. I don’t think anybody has made a decision as to how to take these model outcomes and use them for any type of specific actions that will be of utility and changing the outcomes.
Even prior to this, there used to be these challenges for predicting when the peak of flu would. Although useful in terms of generating improvements and models and predictive power, I always questioned what the hospitals were going to do about this data.
Hospitals weren’t listening to this data: they weren’t changing their behavior and they weren’t changing their operations. What was the utility of that? That’s one of those big questions that we need to answer outside of modelling but more as a societal question: how do we deal with what are the predictions of models?
Erta Kalanxhi
Great, thank you Eili. Very interesting description of forecasting and behavior. Thank you.
We are now moving on to our next speaker, Dr. Brian Wahl. Brian, throughout the COVID-19 pandemic modelers from many other fields, including physics, have attempted to model diseases. How was the field of epidemiology benefited from these contributions?
Brian Wahl
First of all, thank you for having me. I think it’s important to note upfront, as Eili was mentioning, that mathematical modelling and epidemiology has benefited tremendously from other scientific domains.
The law of mass action from physics and chemistry really forms the basis of our standard compartmental model: the SIR models that many of us have been using to model the spread of COVID-19 around the world.
Cross pollination of ideas and methods is incredibly important and is fundamental to the scientific process. There have been many ways in which modelling has benefited from other domains: physicists in particular, but also mathematicians, and several other domains, such as computer scientists who have engaged in mathematical modelling around COVID-19.
There are also the not so good aspects of it, and I’ll maybe describe some of the limitations that I’ve observed as well.
One important component is that there were many models that were developed, especially in the early days of the pandemic. It wasn’t the absolute number of models that was a contribution in and of itself, but what we did see was that the best performing models seemed to be the ensemble models.
There were a lot of examples from the US in which ensemble models eventually provided at least two to three or so weeks out – the best estimates of the trajectory of the epidemic. In many settings, those ensemble models may not have been possible without the contribution of models that were developed by physicists and mathematicians, and so on and so forth.
I have been surprised that people find surprising that not all epidemiologists are mathematical modelers. I know many more epidemiologists who are not mathematical modelers than who actually are mathematical modelers. I have seen a number of examples of physicists in particular, but also mathematicians and epidemiologic modelers, working with epidemiologists who don’t have expertise in modelling to develop models.
I’ll give an example from some of the work that we did in India: I do mathematical modelling, but also collaborate with physicists and developed a mathematical model to look at optimizing vaccine introduction strategies in India.
I also looked at school reopening, using models that I don’t normally work with and working with colleagues who work more readily in, for example, agent-based models. We were able to address some of these key questions related to school reopening.
Both Chadi and Eili mentioned some of the integration of, for example, fluid dynamics into models. Physicists are really well positioned to describe some of those properties and working with epidemiologists, integrating those into existing or new mathematical models as well. I think that’s been an important contribution.
I would end by saying that it hasn’t been completely smooth. There are examples of non-epidemiologists engaging in mathematical modelling that that may have furrowed the brows of some epidemiologists. That’s not to say that epidemiologist also didn’t develop models that were not useful, but there were a couple of things that I found interesting.
The balance between getting, say for example, you’re developing a compartmental model, developing these highly complex models with numerous complex compartments, striking a balance between that and a more parsimonious model that has strong assumptions.
I think I saw tension in that balance among modelling efforts where epidemiologists were not fully engaged. Other epidemiologists may have also experienced this, this kind of rediscovering of results that epidemiologists have maybe known for years.
There are examples from my work where someone who had developed a model came to me with this very exciting finding of how to estimate a vaccine related parameter. We don’t need medical models to complex mathematical models to estimate that- we can estimate a bit more directly.
There have been some entertaining aspects of this as well. But overall, if there’s a silver lining in the pandemic, multidisciplinary teams working together to describe transmission dynamics of infectious diseases would definitely be one of them. I’m hopeful that that that this collaboration, between fields that may have been seen as being disparate, continues looking at other infectious diseases as well.
Erta Kalanxhi
Thank you, Brian. I have one last question for you on the future of epidemiology with more advanced tools from machine learning and artificial intelligence.
Brian Wahl
This is a great question and there have been examples of machine learning being used in models for parameter estimation. For example, in COVID transmission models. I’m sure we’ll see more and more of this in years to come.
But I want to go back to the point that Eili was making and what I was describing just previously: this tradeoff between parsimonious model and a simple model with strong assumptions that can give the right answers to a question that maybe you’re trying to ask. This comes back to what is the question that you’re trying to answer?
Elli was making a really strong point that we think of mathematical modelling at many times with prediction models and looking two to two or so three weeks out in the case of COVID, but there are examples of mathematical modelling where qualitative answers can also be incredibly helpful. Thinking through how appropriate tools, whether it’s machine learning, artificial intelligence, or otherwise, are really tailored to the questions that you’re trying to address.
Ultimately, I think beyond even mathematical modelling, I think there are a lot of opportunities for advanced computational methods – AI and so forth to advance the field of epidemiology.
I think there are also challenges that need to be taking into account as well. We can use AI and machine learning in causal hypothesis testing, but there are, of course, risks associated with identifying spurious relationships or relationships that are not necessarily meaningful from a clinical or a public health perspective, but maybe because of statistical power or the kinds of tools that are being used, are identified as being causally linked.
This speaks to the importance of strong epidemiologic training and epidemiologic reasoning and collaboration between those who are working to develop AI tools and epidemiologists.
I can quickly share one slide from some work that I’ve done previously. This is from a paper called “Artificial intelligence and the future of global health”, and we looked along the entire value chain, from development of AI tools to deployment. The need for user driven research agendas that align with digital principles that have been developed in consensus with experts from multiple fields, but also with guidelines on statistical, ethical, and regulatory standards as well.
We’re in a at the frontier of using AI in epidemiology and in global health. More and more of having some of these standards in place is going to be really important for ensuring that the field benefits most greatly from these new developments.
Erta Kalanxhi
Thank you very much, Brian. We’re now going to move on and talk a little bit about COVID modelling in the context of health policy. We have a couple of questions for Professor Sheetal University of Cape Town.
One of the challenges with the COVID-19 pandemic, which was mentioned today by all the speakers, is the issue of not fully understanding the self and the parameters associated with specific characteristics. Given those challenges, forecasting will result into large uncertainty bounds. How can modelers effectively convey these uncertainties to policymakers without distorting the public health messaging or without undermining the useful of these models?
Sheetal Silal
Firstly, thanks so much for inviting me to be on this panel today. I’m going to share my screen with a few supporting slides.
My involvement in the COVID pandemic has been from the perspective of supporting policy and primarily for that reason and that purpose. All the models that we developed were particularly to be answering questions on the nature of the of the epidemic as it evolved in South Africa, variant after variant, within without vaccines, and then to support decision making at a very large scale.
On the smaller side, it was about preparing hospitals from the point of ordering drugs, having enough staff at each hospital, and having enough beds, all the way up to at the presidential level of making national policies on lockdowns and curfews and so on.
When the South African COVID-19 modelling consortium was founded, which is a group of modelling units who have come to together from a variety of universities in the country, we had to make a decision on the kinds of modelling that we would provide.
To answer your question directly, we did not attempt to forecast for very long periods. We rather chose to do short term forecasting, but then use a scenario approach to our long term models. We knew that in order to be most effective in decision making, we needed to make long term projections at a time when almost nothing was known of COVID.
That is why I have labelled here things as the knowns, the unknowns, and the unknowable. Many aspects, even four waves into COVID, of the disease, population, behavior, and response to restrictions and so on is unknowable.
Certain unknowns have become knowns or better understood throughout the epidemic, but what that means for us and how it works towards gaining and maintaining public trust and maintaining government trust of your models, so that your modeling evidence can be a key input in decision making, is through constant adaptation.
First, we have a variety of models. We use statistical models with confidence bands and do short term forecasting at the admin level one granularity. By forecasting cases and hospital beds say two weeks into the future, you’re giving an idea of immediately what’s going to happen.
Short term forecasts will tend to have smaller uncertainty bands and be better performing than trying to figure out what is going to happen in six months’ time, when we have absolutely no idea as to the emergence of variants and population behavior, and so on.
With the long-term forecasting, we adapt our compartmental models time and time again to take into account new strains and so on, each time generating a set of scenarios where you can gain confidence by showing that our models are adequately capturing the data in the background, and then providing a set of scenarios as to based on certain assumptions, what the future might hold over the next couple of months.
Then you gain public trust, and for us it was honestly more important to have government trust, by repeated presentations, repeated engagements, and almost a constant set of meeting going on to describe your models, describe your changes, and the assumptions that you have to make.
Moving forward from some of the discussions or some of the points raised by the previous speakers, we have learned in our experience of modelling, not just in COVID but throughout modeling of all diseases, that mathematical modelling is not the domain of the modelers alone, but it is multidisciplinary. In our modelling consortium, for example, we validate our projections with epidemiologists and in the manner of clinicians, intensivists, virologists, but also the public health specialists, as well as the economists.
We feed our modeling results into macroeconomic models and so on, so then what we’re able to do is provide evidence on the whole system in which the disease’s actually transmitting. In the context of modelling for an LMIC, like South Africa and other LMICs that we’ve worked in, you have to take into account the context and the issues with the data: we cannot just accept whether it is big data or small data or any matter in between.
To be able to understand the underlying data structures and the flaws in the data is vital to contextualizing your model output.
That helps in gaining the trust and the buy in from your government partners and the public, when they can see that even though you may not have the most complex models – for us we had compartmental models not agent-based models simply because a reliable data did not exist to train agent based models in country – they are aware of certain heterogeneity is not being captured.
By contextualizing your results from a simpler or less complex model, you can regain the trust of the public and continue to have their interest in the future updates of your model that you’re providing.
Erta Kalanxhi
Thank you very much for those insights, they are very interesting. We have another two-part question for you. In what ways do models and statistics address current health inequalities, and do logarithmic biases in artificial intelligence exacerbate the inequality gaps?
Sheetal Silal
I was recently participating in a WHO consultation on exactly this topic. There was a discussion on if the datasets exist for models to take into account these heterogeneities and these health inequalities that are particularly relevant in LMIC.
One of the outcomes that came out of that meeting was that it is not always necessary to directly include this data into models in the form of further stratification between income groups, for example, because there are challenges with having adequate data to train your model for all these levels of heterogeneity that you would like to include.
Being able to contextualize your findings for a particular area, with respect to aspects of health inequalities like demographic differences and historical differences in how data have been captured.
I come from South Africa and for many black or non-white population, historical data does not exist or data from certain years are simply unreliable because it excludes large parts of the population. Likewise, taking into account access to health care: you may have predicted that we anticipate admissions in one area to be at level x, but actually if the population does not have much access to health care, you’re going to see much lower admissions in that particular geographic area.
You could also find higher deaths when you measure excess mortality – deaths occurring from disease both in and outside hospital. Being able to contextualize these findings and understanding again how bias is in the data and how the data are collected exist, these are other ways in which models can help to address health inequalities instead of exacerbating them.
I think what is key to something like that is making sure that the people doing the models for an area are empathetic and have that contextual understanding of the area for which they are modelling. Otherwise, this level of knowledge is often missing.
To address the second part of your question on the use of AI exacerbating health inequalities, I think that whether it will be big data or any other size of data, the blind use of data and blind interpretation of any model without contextualization can result in negative outcome whether you use AI to develop your models or use any other methods.
Factors of health inequalities, like disparities in living and working conditions and access to healthcare and systemic racism, all can lead to a disproportionate level of vulnerability to disease. These are not always uncovered or able to be understood in large datasets or by our algorithms that we seek to implement.
We can have methods that rely purely on data for predictive capabilities, actually exacerbating healthcare inequalities, but that doesn’t mean that that the methods are not suitable. Models shouldn’t be divorced from modelers and shouldn’t be divorced from the content and the population for whom they are being developed. I think that is to key to minimizing healthcare inequalities
Erta Kalanxhi
Thank you very much for the insights, very interesting discussion with practical consequences for what we are experiencing today. We have reached the end of the hour. Thank you very much for all your insights.
I was just going to ask the audience if they have any questions for our speakers. It is the time to enter them now, either on the message in the chat or the Q&A section. In the meantime, if you have any closing remarks or any comments that you’d like to make, our audience is still there. We haven’t been abandoned, but we just haven’t had any questions. Probably everybody’s captivated with all your discussion.
Eili Klein
There is a question about the forecasting future. I think that’s what everyone wants to know, when is this going to end?
Since the day that they closed —-. I actually was in Portugal at the time and I told my wife that we’re in trouble now. Once you close a city of 20 million it’s already too late- it’s already escaped, and we’re going to have a lot of trouble.
There’s a lot of twist and turns and a lot of uncertainty, obviously, but what we’ve seen is a virus that has behaved in many ways similar to what we would have expected in a general approach. If you look at the average over time, things have generally done what we would have expected. The local level of things has been very uncertain.
When you look at the what Coronavirus has done historically, they have generally become these very rapidly evolving viruses that cause not generally significant disease. The big question that I have been asking for a while has been whether or not this is going to be moving the same way most Corona viruses have, which is towards a not a particularly dangerous virus that rapidly mutates and causes waves of infection every year, but we don’t really generally notice or track it at a population level.
Nobody’s talking about it on the news. Or is is going to be more like the flu, where it is causing severe disease to the point where we actually want to vaccinate against that on a yearly basis? Those are sort of the two more likely outcomes.
Given Omicron, where it does seem to be spreading faster, but potentially causing less severe disease, unclear what that means for people who are unvaccinated, but it looks like it’s reinfecting people who have already been infected previously.
We’re going to continue to see waves of this and the real question is that is this going to cause mild disease that we’re going to just live with, like the common cold, or is this going to be something where we need to continually update the vaccines and constantly vaccinate every year like the flu? I don’t know that the future is certain on that.
I would tend to believe that this is going to behave more like what we historically see with other coronaviruses, that it’ll move towards a less virulent pathogen that causes continual waves of disease but not particularly dangerous.
Erta Kalanxhi
I just have a quick question, perhaps a concluding question. At the beginning of the pandemic, it was challenging because it was not a lot known about COVID-19. Now the challenge is that we know a lot about the variants, different variants. Is this an easier task to deal with then what we dealt with at the beginning? Are we better off now with all this information that we have?
Sheetal Silal
As challenging as it seemed, in the beginning, I feel like in retrospect, it was an easier time than now. In the absence of any data, you make a bunch of assumptions that you hope are reasonable and perhaps look to extremes and you can have a few scenarios of the future.
Once we have all of this data, we now need to be able to unpack where conflicting outcomes are and what are the most plausible- what are suitable to your setting. I think it was Eili who raised the issue of mobility data. In wave one, we also brought in tons of mobility data. It was wonderful and it helped with assessing movement within the country.
But at the end of wave one, it was literally useless. You have got to be able to unpack these data sets, and all that takes a lot more time then back in wave one, where it was fine to have quite simple models and make a bunch of assumptions and spending a lot of time understanding the where, why, and who your data actually represents. That will be my perspective.
Erta Kalanxhi
Thank you, Sheetal. We have one last question. What is the required background to become a modeler in epidemiology? I think that Brian talked a little bit about that. It’s a nice way to end the webinar for everyone who is aspiring to be involved in modeling.
Brian Wahl
I think anybody could provide their insights on this as well. I think COVID has kind of changed things in terms of mathematical modelling and epidemiology. I’ve seen, as I was mentioning, interdisciplinary teams working together on mathematical models. I think there could be many paths to being involved in work as a as a modeler, either directly or indirectly as well.
I would hesitate to say this is the one path that you need to take in order to become a mathematical modeler. Interdisciplinary teams are working together and there are several paths. That may not be satisfactory, but getting involved in work that’s ongoing, studying quantitative training, whether it’s mathematics or physics or other fields, could provide a strong foundation for getting into mathematical modelling.
I’d be interested to hear others on the panel and the path that they took to get here and how they see that maybe changing in the era of COVID.
Eili Klein
I probably have the strangest path. Out of college, I worked in IT for a while doing web development and things like that. I then went back and studied health policy and somehow landed a job with Ramanan. I was working on epidemiology type of projects.
This colleague of Ramanan came in, David Smith, and he was about to go on paternity leave because his wife was about to have twins. He had some models that had been he finished, so he dumped everything he knew about malaria modelling onto me and said, here you go. I didn’t see him for four months and I just had to figure it out.
I knew how to program and I had a background in math. Anybody can call themselves a modeler, that’s not the point. I think the question is, what do you want to do with becoming a modeler in epidemiology?
Brian’s point is that there’s a lot of things that go on, and many of them are now interdisciplinary. Where do you want to find yourself in 5 or 10 years? Do you want to be in academia, in which case you probably want to be in an academic setting? Do you want to be in government policy, and helping to interpret models and drive policy, then that’s a different pathway that you might want to follow?
I think that it’s more like, what you want to do with anything that you study and learn about, whether it’s modelling or epidemiology or medicine. There are people who go to medical school, but don’t want to be doctors in the same context. It’s really about what you want to do with the concept of modeling and, as I said, modeling can be useful for population level modelling.
When we talk about SIR models, that’s a lot of what we’re talking about and a lot of what COVID-19 is. You get a lot of news and press about it, about the sort of larger models that are trying to predict how people are going to how the disease is going to spread through the population.
That’s not the only modeling that there is in epidemiology. There are people who model at the chemical level, people model at the cellular level. How does cancer spread in the body or how does neurological model spread?
There’s even all the AI stuff. On the side, what I would love to do, is work on the concept of how neurons actually work, which is different than exactly the way AI is built. It’s built on that concept.
There are people who are working on those types of things, which might have both biological importance as well as relevant economic and other factors. This may not be exactly what you’re defining in terms of epidemiology, but epidemiology and Ecology and Evolutionary Biology are all large broad concepts in which you can do a lot of interesting things.
Erta Kalanxhi
Very interesting, thank you so much Eili for sharing this with us. I would like to thank everybody for sticking around until now. We’re 10 minutes over original time that we had planned. Thank you very much to all of you for joining us and for sharing your views. I know these are busy times, as I said at the beginning, so we’re really appreciate you joining and giving us such interesting insights on this very, very relevant topic right now.
COVID-19 has made modeling very, very popular at the moment. Me, not being a modeler myself, have gotten educated at some point by working with Eili and others. Thank you very much. Have a great day. We’ll see you on our last webinar, which will be sometime in 2022. Thank you.