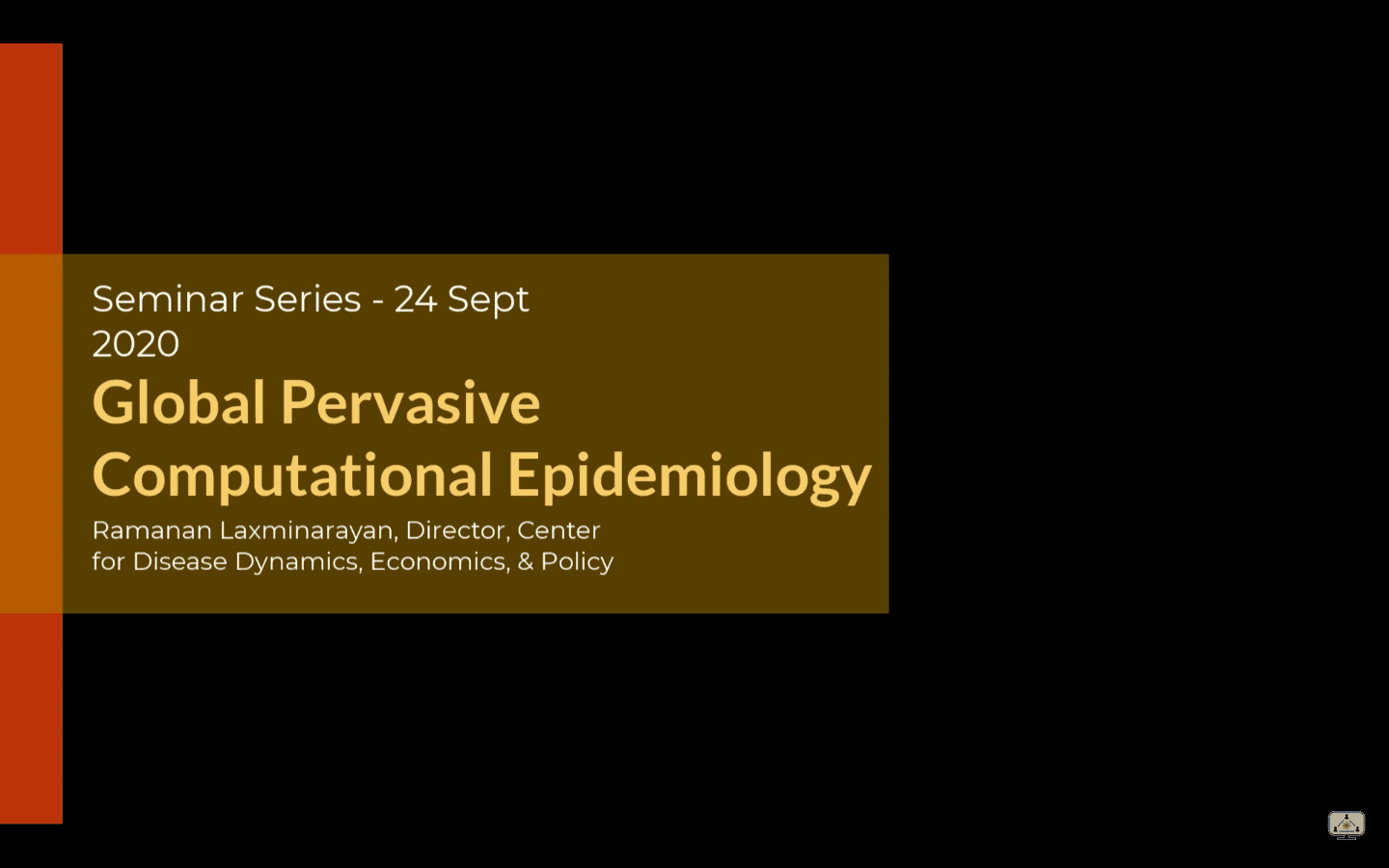
You can also view a recording of this webinar here.
Golda Barrow
I want to welcome everybody to our GPC Seminar. Our speaker today is Ramanan Laxminarayan. He is the founder and director of the Center for Disease Dynamics, Economics and Policy, and member of our Expeditions Program team, so welcome. Thanks Ramanan, I’ll turn it over to you.
Ramanan Laxminarayan
Okay, great. Thanks everyone, thanks Golda and Madhav. So there’s no mathematical modeling per se in this paper, but I think it’s a useful complement. Early on in the epidemic, we did do a lot of mathematical modeling when we didn’t have the data. Now we have so much data that we’re trying to catch up on this, and then we’ll model after we go forward from here.
As many of you know, India has got a fast-rising epidemic compared to other countries, where the epidemic has waves or things coming down after a period of time — hasn’t happened in India per se. Daily reported cases are between 80 and 100,000 cases, or 110,000 cases, in that range, and there’s a lot of underreporting.
Even with that, the cumulative death count — these are a bit outdated because this was for a perspective I wrote for the American Journal of [Tropical] Medicine — August versus 40,000, today that number is about 90,000 deaths. So India is gaining on the US quite fast, it’s already #3 after Brazil and the US, but I have no doubt it will catch up if the numbers are counted correctly. It’s possible that India has a bigger epidemic already than the US.
The number of tests conducted has also been increasing, but even so, India ranks about #111 on the ranking for tests per capita, it’s about in the 80s for both cases and deaths per capita. So clearly, there’s a lot that’s hidden about the epidemic. There was a lockdown that was imposed very early in India on March 24th at midnight with four hours notice. Pretty much the whole country was shut down, all transport, road, air, rail. I don’t think the railways have ever been shut down like this since they were introduced in the colonial times so this was quite unusual and everyone was asked to shelter in place.
There was a second period in April, Phase 2, from 15th April to 3rd May, when there was some amount of interstate movement of stranded people that was allowed, but still by and large the place was shut down. Third phase, again, some additional movement and fourth phase as well, but even today, although the national lockdown has been lifted, there are still many state lockdowns which are in place. So you can see, local trains are now allowed, but India has had the most stringent shutdowns anywhere in the world and [it’s] hard to imagine a country of that size to be able to carry out something like this, but it really hasn’t stopped the disease. It slowed it down, but it hasn’t stopped it.
So the first round was called the Lockdown Phases, and the second ones are all the Unlock Phases. But even now, the fear of the disease is high enough that you won’t see a lot of people just wandering around in urban areas, certainly in rural areas it might be different.
This study is based on data from two states: Tamil Nadu and Andhra Pradesh, these are pretty large states, roughly about 70, 80 million people each, and Andhra Pradesh is a bigger state than Tamil Nadu but it used to be part of an even bigger state, which was called Andhra Pradesh, and then it split into Telangana and AP.
So the top part here is AP, this is the coast over here, and this is Tamil Nadu below. These are some of the urban centers which are marked over here and this is the change in cases per 10,000 going from 31st May to June into July, and you can see that new cases have been climbing rapidly. This is again cases per 10,000 by district in AP and Tamil Nadu and it’s actually interesting, because these are two states with reasonably good public health systems, so the ability to track cases here is much better than the rest of the country.
Now we had looked to see the other contact tracing studies and, in fact, this morning I had someone pull it out, and the largest study to date, which is on household secondary attack rates based on contact tracing, but this study which was in The Lancet a few months back, they had 215 primary cases and 195 contacts that were traced from those. We haven’t really been able to find many larger studies. The last one is also on COVID, the remaining are not even from COVID.
So what we have is a bigger sample. We have 435,539 cases, we’re tracking about 5,700 deaths. We overall had [5]75,000 contacts from about 85,000 primary cases. So clearly, much larger than any other COVID study to date, and certainly than any other infectious disease study to date. When you really break it down, the contact tracing in these states was just mind-boggling. I’ve talked to people here, and I don’t know if there are data sets of this kind available even in the US, and of course, in sheer numbers, India’s going to be larger, but I don’t know if the contact tracing effort was that great.
The one thing about India was that contact tracing was initiated very early, it’s one of the bright spots in the public health response to the disease. So it reached about 3 million known exposed contacts, confirmed cases, by August 1st [2020] itself, and I’m just talking about these two states. And then [at] the individual level, data were available from about 575,000 contacts, from 85,000 confirmed cases. We really look at it as that’s the total.
In the first version of the paper we had plenty from both, but after some time, the government of Tamil Nadu only started reporting aggregate numbers, and these are all the data from the states, so these are not in the public domain, but AP continued to do it. It’s possible that we might get the same data from Tamil Nadu as well, which would make it about a million contacts, but we were just trying to get the paper done and published, so we didn’t go after getting that entire thing done, it would have taken us another month to sort that out.
Just to finish there, like I said, about 1.8 million contacts tested, so all of these folks are subject to, in these two states, RT-PCR mostly in Tamil Nadu and [in] Andhra Pradesh, about a 60:40 ratio between rapid antigen tests and RT-PCR tests. So we had the known test outcomes for this number, and we still have this information. But we didn’t have the epidemiological data for the entire lot.
Now traced contacts tended to be younger and were more often female than their linked index cases. The test positive individuals identified were about 1.3 years younger and less likely to be male than the overall population of the two states. So these are the attributes of the cases and the tested contacts. I apologize, a lot of it is just descriptive epi[demiology] at this stage because we’re grappling with the numbers here, but we do have some results in terms of takeaways as well.
This is exactly what I said in the previous slides, which is that the index cases are far more likely to be in this under-50 age group, not so much in the older age groups. More in AP, but as a proportion, still lower because as you can see, the numbers are all large in these index case groups, I guess because they are the ones that pop up for having transmitted to other folks. The contacts also tend to be in the younger age groups.
If you compare the index cases with contacts tested and test positive contacts, and I have this as a figure as well, you can see that the reported cases without contacts tested, there are still about 350,000 and then test positive trace contacts, about 42,900, and more likely male in both of these categories. And again, like I said, the bulk are in these middle categories. Actually, we say “male” and “female,” but we actually have some transgender, India does record transgender, so it is an acceptable criterion in the way gender is recorded.
We also had data on the criteria for the contact. The way this works is they find someone who is positive, and then they say, okay, you’re positive now, who all did you come in contact with? And then they classify the contacts into two categories: a high risk criteria is one of these, they touch the bodily fluids of the index case or they came into direct physical contact or they touched their linens or live in the same household, very close proximity. The low risk criteria was when they just shared the same room perhaps, or they were in the same bus, but they could have been sitting in the first seat of the bus and this other guy could have been in the last seat of the bus, so the low risk criteria truly are much less likely to be infected.
The daily cases by state, Andhra Pradesh is in the purple, Tamil Nadu is in the blue, and Tamil Nadu had an earlier epidemic than AP because it has more international traffic coming in. Although the testing ramped up in both places in the same wave, the daily caseload was much higher in Tamil Nadu initially, and then Andhra Pradesh caught up, and similarly for the deaths as well, which obviously lag the case data. This is the cumulative incidence and mortality by state and it obviously doesn’t show any signs of going down at all, these are all headed up all the way through to, our data go into about late August at this point in time.
This is the distribution of index cases by contact age. It is in the tables that I showed you before, but it’s just a little easier to see in the pictures. You can see, this is the contact age, and this is the index case, so we’re picking up a lot of young people, primarily because these are kids living in the same household as the index case. And this is the distribution of contact age cases by index case age, just flipping it around that way. So this is the contact age of the young, then the index case age was likely to be in that [area].
Madhav Marathe
Ramanan, can I ask a quick question?
Ramanan Laxminarayan
Yeah, yeah.
Madhav Marathe
[Was the index case] picked up from a previous contact reporting?
Ramanan Laxminarayan
Could have been, could have been. It could have been picked up from a previous contact reporting as well. I mean, that’s how they do it. They were not doing this in a study mode, they just said, listen, if you test positive, I’m going to find out all the people you were in contact with. It seems mind-boggling for a country the size of India, that they would go and try to do it, but I visited my mother two weeks ago in Chennai and I was there just for two days and both days, a girl would come in the morning from the public health department and check to see if she’d had any sort of symptoms. I mean, this is for one 83-year-old living in one place, and they go and check that way.
So I have to say, these states have not done poorly because of lack of effort. The effort that they put in is incredible. It is the population and the size. You’re talking about these states put together, about 150 million people, about half the size of the United States, the total data that I’m showing. I mean, they represent half of the population of the United States.
So these are the same pictures, but broken down by high-risk contact. You can see, [it’s] roughly similar. The high risk contacts are very similar in terms of the overall contact. This is not exactly the same thing, this is a distribution of index age by infected contact, so here (previous slide), the only difference is it’s by contact, here (current slide) we’re seeing if the contact is actually infected. You’ll see this now more in the later pictures, we see that once you start getting to infected contacts, you’ll see a lot of this kind of a 45 degree line, which is you’ll see that a lot of the transmission has happened between people who are of similar ages. Here again, if you have an infected contact, chances are that they’re index case was in that middle-age category, but if you look at the distribution of high-risk again, you see the same thing as above, which is you’ll see that the infected contact age is highly correlated with the index case age. Generally, people tend to be giving the disease to other people of their same age group.
We have secondary attack rates as well by interaction type and setting, so you can see we have any setting and then we have community, household, travel together, and healthcare, and the secondary attack rate is highest for people who travel together. For any contact, similarly for high-risk contact as well, lower [secondary attack rate] for people who are in the household and then lowest, of course, in the community. None of this should be surprising in a sense of what we now know from many studies. The reason why it’s interesting here is because it comes from such a large dataset, so we have a much better handle on it and therefore there’s more confidence.
Madhav Marathe
So the household part seems more surprising, Ramanan, right? Travel, clearly, but household people are in very close contact, especially in very small houses and places, so why is that number so low?
Ramanan Laxminarayan
Not really clear. Because the Chinese clamped down so fast, most of their secondary attack rates came through people in the same household. I don’t know what to make of it, because we don’t have a lot of things to really compare with in a sense, but these secondary attack rates are not different from what we’ve seen from other studies for within the same household [rates]. But I’d love to hear from other folks in the group if they’ve seen something much different, but this number’s not that different, a 1 in 10 for a household transmission. The Chinese studies, which are the only other ones that I’ve seen, are not very dissimilar. I’ve not come across a whole lot of papers like this out of the US, for instance.
Madhav Marathe
So intuitively, just because you fell sick at home, it’s not like your entire household was sick?
Ramanan Laxminarayan
No, no.
Madhav Marathe
I mean this is somewhat surprising to me, given that the houses are densely packed, as you know.
Ramanan Laxminarayan
It is, it is. But if you start looking again at the…so, when you get into the superspreading data that we have, it’s also not clear that everybody is spreading easily. Obviously, superspreading is a function of both the setting as well as the individual’s shedding of the virus, but you’ll see, surprisingly, that something like 10 percent of people are responsible for 85 percent of the secondary infected cases. It’s highly skewed in that way. Let’s come back to that point, let’s park it, and it is a good point, but I don’t think we have a different rate than other studies indicated. I think Chinese households are not very different from Indian households in terms of size, like in Wuhan, I don’t think they have huge houses.
Madhav Marathe
I would think they are slightly different. The big difference is younger kids at home, and maybe they don’t pick it up. But yeah, we’ll table it so we can let you finish.
Ramanan Laxminarayan
The contact distribution, I forget the median here, but it’s something like 10 or 15, if I remember right, but basically, you can see that they went all the way to 80+ contacts of an index case. It was mind-boggling. The total contacts positive obviously was going to be a smaller number. You can see that at about the 72 percent mark, you really don’t have — these are the index case percentiles — there are not many secondary cases. This indicates that starting at about the 92nd percentile upwards accounts for 60 percent of the cases. Eight percent of the index cases account for roughly 60 percent of the positive contacts. It was more stark in the early stages of the epidemic.
We did this analysis twice, once when we had the paper, we had it accepted, and then we said, we have more data so give us two, three more weeks to do it. They were kind enough to let us revise the paper entirely. By that time, all our numbers had gone up by 10x from when we had submitted. So we had a more stark superspreading finding in the first round, it’s still pretty stark but it’s not as it was in the first round.
This is for case and contact sex so you can see there the probability of a positive contact percentage is higher for the female-to-female and lower for the female-to-male. The low-risk contacts, it’s pretty low, and it’s at about 4.5%. To me, that’s still quite high, but yeah, it’s about 12% for high-risk contacts.
This is interesting, again, I’ll show this to you in a picture, but these are contacts and the positivity rate…these are the index and this is the positivity rate, so the x-axis is index age and the y-axis is the positive percentage in contacts aged zero to four. Let’s start from here. Index age of the kids, high positivity rate in the contacts of zero to four [years of] age group category. If you work your way across the figures, you can see that there’s a lot of this age cohorting effect, which is that kids are giving the disease to kids and the elderly are giving the disease to the elderly. You can see that better in this picture, actually. Look at transmission probability by age given exposure for all contacts, and this is the contact age, that’s the index case age, and so the dark red is 50 percent and the lighter pinks are somewhere between 5 and 20 percent.
You can see this is a very strong 45 degree line that shows up here, which seems to suggest this age cohorting effect, and certainly in the high-risk contacts particularly, you see that kids are transmitting a lot to the other kids, which is…there’s been an open question in the literature about how important a role kids play in COVID. I think, at least from this dataset, it seems to indicate that they do transmit a huge amount, going by, cutting it in all different ways.
Madhav Marathe
Ramanan, actually something more interesting also is that kids are also giving it to their parents or people in their home, that’s a really interesting pattern.
Ramanan Laxminarayan
That’s what I meant, that kids are playing a strong role in the transmission.
Madhav Marathe
There is an age-to-age sort of activity, but there’s also small kids to parents getting it in the family. Yes, interesting.
Ramanan Laxminarayan
Absolutely.
Predictors of time to death…this is the second set of results which are around predictors of time to death. As we see in other studies, the elderly are most likely to be there, then males about 62 percent higher, then of course, you see this interesting effect where, as time goes by, people are less likely to die possibly because of earlier detection or better medical care. Tamil Nadu has a slightly high risk of dying and these are all adjusted hazard ratios. The reference is 50-64, but you still see a lot of people in this age group, which is 65-74, and 75-84, it’s not just the >85 year olds that are dying.
These are case fatality rates. What is unusual here is that I actually wanted to put up a direct comparison to the US, I didn’t have time to do that, but the case fatality ratios are actually pretty high for the 55-64 age group compared to other countries. Also, for the 65-74 age group. This 16.6% is not unusual given that first Wuhan study and everything that came after that. What is unusual about India is that these age groups, 50-84, are much higher, it appears, in India than it would be in other countries, at least in the developed countries. We don’t have much comparison data from developing countries, but I’m sure you all recognize that these are high case fatality ratios compared to the studies from China and then also from Western Europe.
Madhav Marathe
Is this conditional on the fact that you got the disease, or…?
Ramanan Laxminarayan
This is conditional on the fact that you got the disease, yeah. So males is obviously higher than females, the ratios seem higher than what, I mean, in a tight paper like this, you could devote exactly one sentence to discussing this, but the ratio seems much higher than in the other countries where these studies have been done.
This is what the survival probabilities look like. Basically, about half die by day 6 or so. The slope of the curve is such that it’s more stark for men than women, the women are slightly more uniform. The median time to death here is about 6.5 days, if I remember right. So it’s about 6.5 days and again, males are actually roughly in that, slightly less and women are slightly more in terms of median [time to] death.
Now, just for comparison, the US median time to death is about 10 days, I think, it’s more than that, there’s greater survival. If you look at population age distribution, we’re just comparing this to the US because this is the data we had as a comparator, if you look at India versus…first of all, India itself is different in different parts of the country. Andhra Pradesh and Tamil Nadu are wealthier states in general, they’re also older states from a population age distribution standpoint than the northern states of UP (Uttar Pradesh) and Bihar, which account for something like one-third of the births in India, just from those two states.
The elderly population across India above 65 [years old] accounts for about 6.5% of the Indian population. In comparison, that proportion is 19% in the UK and 22% in Italy. So, this has been the hypothesis about whether India as a whole has a lower mortality rate because of the younger population, but that’s not a clear story because much of the mortality that has been observed has really been in southern and western India, where that skew is not as great. That younger population is mostly in these poorer states of Bihar, UP, Rajasthan, for instance, and not throughout the country.
If you look at this population age distribution, it’s younger in India but not hugely younger than the US. I mean, it’s somewhat. But if you look at the reported case distribution as well, it’s again, not super out of sync compared to the death age distribution, which you can see, India’s remarkably younger in terms of age of death compared to the US population. This is a bit perplexing, I don’t think we have a clear answer why, but this is what we’ve observed. Like I said, again, this is far more of a descriptive epi[demiology] paper than really something which can disentangle reasons for all of these.
Last but not least, there have been early questions about whether this lockdown that was imposed in India was really of any use and what we see was the effective reproductive number was quite high. It was in the 2-3 range and around roughly that, or even higher, in AP. The lockdown did have an effect of knocking it down quite significantly. Tamil Nadu, in fairness, their Unlock has been harsher in the sense that they’ve not unlocked a whole lot. AP did unlock more, and therefore you see a bump up here, but this is what we observed.
Just to close, I think our main takeaways are, risk of transmission from an index case to a close contact ranges from about 2% in the community to about 9% in the household, and doesn’t really differ with respect to the age of the index case, not a huge amount. Kids and young adults have an important role in transmission and one-third of the cases are under the age of 30. Superspreading events seem to be the rule rather than the exception, so the prospective testing of the exposed contacts found that 83% of infected…actually, these numbers have slightly changed, so this number is slightly lower, but you still get the idea that a small number of infected individuals accounted for the vast majority of observed new infections.
This is the largest empirical demonstration of superspreading that we’re aware of in any infectious disease. Unlike in developed countries, the deaths are mostly in the 50-64 age group, and the incidence of reported cases also doesn’t increase with older age. We can’t justify this fully with differences in the population age distribution, so clearly [there’s] something else going on.
Then, contrary to long hospital stays, our median time to death was 6.5 days following admission.
And finally, we did see a substantial reduction in the reproduction rate associated with the country-wide shutdown. This has been hypothesized, but not really shown empirically before, so that’s really what we were after.
As you can imagine, it’s a paper, but it’s really the work of thousands, if not tens of thousands, of public health workers in Tamil Nadu and AP who got the data, and then it was put together, and we had tremendous support from these two state governments in carrying out this analysis. And Joe Lewnard did much of the heavy lifting here. That’s pretty much it.
Madhav Marathe
Thanks Ramanan, excellent, excellent talk. I have many, many questions, but I’m going to open it up, I’m going to let others ask because I think it’s only fair that they get a chance. I’ll let Golda run the Q&A session from here.
Golda Barrow
Sure, we had a few questions pop up in the chat. Kathleen Alexander?
Kathleen Alexander
Well first, I want to say, what a great talk, really spectacular, and I wish we had that kind of data from other places, wow.
First of all, I thought that was so interesting, the travel versus the household infection rates, and I wondered if you would mind sharing a little bit about how they define a household member, because it can be so fluid and whether those sort of transitory connections may or may not influence that.
And then, secondly, I’m sorry if I missed it, but the characteristics that you thought were most critical or potentially could be explored for the superspreading events, that’s really cool, that kind of an idea that there is this really core group of folks, and what is it about them, if you had to speculate, that you feel the data suggests might be characteristic?
And then lastly, more just a question of how much you believe the HIV contact tracing infrastructure in human resource development contributed towards this ability…this is amazing what they were able to do, and I’m impressed.
Ramanan Laxminarayan
Let me start with your third question. Without doubt, HIV really helped — I mean, to the extent that HIV helps anything at all — but the whole public health department was really, they were not doing contact tracing for the first time, they knew how to do this. They knew how to do it largely because of TB and HIV. Particularly Tamil Nadu, but also Andhra Pradesh, they’ve had a pretty strong track record of dealing with HIV, so India compared to the projections early on did quite well at controlling HIV. I mean, India was supposed to sort of be the global capital of HIV, as was projected, but I think the work these folks did helped keep it down. So definitely, that played an important role. Thanks for highlighting that.
Madhav Marathe
Ramanan, I want to point out Jim Koopman is online too, and he played a spectacular role in the eradication of diseases in India. I just want to acknowledge his amazing work, his contact tracing work. I just want to let you know.
Jim Koopman
Yeah, it was a long time ago.
Ramanan Laxminarayan
It’s probably been about 15 years or something, Jim, I haven’t seen you in a long time. Hope you’re doing well.
So, yeah. The first question is about households. How a household is defined is, just in Tamil, just a standard, “is there anyone else living here?” I don’t think it’s really a fluidity of household kind of an issue in a major way. I think this was pretty well-said and understood by people.
The second question that you had is really, remember, we’re not talking about superspreading events, we’re talking about superspreading, period. Superspreading being the idea that some people just transmit far more than others, or in those occasions. Because of the lockdown, there weren’t, it wasn’t like people were going to temples or to weddings or anything of that sort. We could look at that, but that was not what we were trying to get at with that picture. We were just trying to show that most of the secondary infections came from the very, very small number of primary cases. Even if you control for the size or setting or whatever else it is, it still seems to be the case that some people seem more likely to transmit than others, and that’s the only point we’re trying to make.
Kathleen Alexander
Nice, nice. Nice work, thank you so much.
Ramanan Laxminarayan
Thanks, Kathy, I have to catch up with you after.
Anuj Mubayi
Thanks, Dr. Laxminarayan, it was an amazing talk. I just wanted to know from the modeling perspective — I’m a modeler, actually — so I want to know, how do you define [contacts]? What is your definition? Because if you change from 6-feet to 7-feet as a distance between [people] or the duration of time, that definition could lead to very drastic results in terms of measurements of your contacts, for example.
Ramanan Laxminarayan
Are you asking how these contacts were identified by people?
Anuj Mubayi
Yeah, so how do they define this definition of the contacts? What does this contact mean for them?
Ramanan Laxminarayan
The public health department, I think I put up that slide which shows the high-risk versus the low-risk contacts, right? This is just a public health definition. They go to the index case and say, okay, now give us a list of all the people who shared either a bed with you, or a bodily fluid, or were in close proximity. They just get a full line list. That’s how they go about it. This is based on those questionnaires, and then they send someone to chase after those questionnaires and call them.
They do this very rigorously, so if you were in a particular bus — I mean, obviously public transport was mostly shut down at that point, so it was hard. It was easier for them to some extent to contact trace, but let’s say somebody is an infected person and then they happen to be on Bus #42 from Place A to Place B, they would go and try to find out who all were on the bus at that particular time.
Obviously, this is imperfect and not always possible, but they tried. They really did try. I think that’s what is impressive about this effort. Really, we’re out here presenting a paper, but kudos to the people who pulled this stuff off.
Anuj Mubayi
Yeah, the reason I’m asking is because if someone is standing at the bus stop and the other person is passing by, very close by, is that considered a contact, or riding on the bus is the only contact for it?
Ramanan Laxminarayan
Riding on the bus is the only contact, so they would just ask who was actually on the bus.
Golda Barrow
We have a really good question from Shankar Basu. He asks, could you [talk about] the mechanism of contact tracing and was the phone app used?
Ramanan Laxminarayan
They did use Aarogya Setu to some extent, but Aarogya Setu is not particularly helpful to the extent that people thought it would be, because for Aarogya Setu to work, you a) need a smartphone, you need to have people using it on a regular basis, and you have to have the bluetooth tracking on. To work Aarogya Setu, you don’t need to put your bluetooth on at all or give them permission.
So most of this was really done through just shoe leather epidemiology. People going out to and talking to other people, they go out with a chart, I say people but they’re often young women in their early 20’s, they show up with their charts, they’re all wearing a mask, and they’ll say, okay, who were you in touch with, and then they go through the list. It’s very old-fashioned, as Kathy said, it’s like HIV, it’s how they did it for HIV.
Golda Barrow
Any more questions for Ramanan?
Madhav Marathe
I have a question, so it has to do with these superspreaders. Do you think that that is an absolute result or it’s because of the sampling bias that might have come. It’s a well-known issue, in fact Chris Tuck has had a nice paper and others have noticed it as well, where you preferentially reach these superspreaders quickly through these nests. Have you looked at it, or [do] you think it’s really the fact that some people just spread much, much more than others? Because if it’s true that it’s an absolute result, then it’s remarkable, it has to be put into a model somehow.
Ramanan Laxminarayan
So, Madhav, there’s no way to tell, right? What are we doing? We’re doing an experiment where you’re saying, “I’m going to talk to 100 people, and then find out who their contacts are, and it’s certainly possible that we are more likely to identify primary cases that were symptomatic just because they present themselves and so forth, so we might not pick up on asymptomatic cases to the extent that we pick up on symptomatic people. Of course, the secondary…what they did was try to test every single contact, especially the high-risk contacts of the primary person, so they did go out and do the testing for the second part, even if they were asymptomatic. That part is taken care of.
The first part, let’s go through the thought experiment. Is it possible that people, there’s some bias in the people that you pick up as your index case. Are you picking up people that are more likely to transmit it to a secondary bunch? Because you’re following that chain, I mean certainly there is bias, that’s possible, but I don’t know how large that could possibly be and what gives me confidence is that we had this in the early stages as a strong result, we still have it as a pretty strong result even though we picked up 10x more data after that. Unless there’s something fundamentally, there’s some bias that we haven’t thought about, I can’t really figure out…what did you have in mind, for instance?
Madhav Marathe
Like the first one because if you do, from a purely graph-theoretic standpoint, I’m actually really amazed by the result, but if you walk in this graph, a random walk, then you’re likely to hit a high degree node much, much more quickly. You’re likely to pick up on these superspreaders.
Then, of course, you test everyone, the probability of reaching a superspreader, a high degree node, is intuitively higher if you do these contact tracing events. But maybe that’s not what happened because I think they changed the final structure. Others on the call should say as well, and I’ll think a little bit more.
But I think it’s a really, really interesting study that you’ve done and we might have a chance to do a control for it a little bit, after we look at the data more carefully.
Kathleen Alexander
Maybe I can just ask, maybe I misunderstand you, Madhav, but I’m kind of intrigued by the idea that, how many of these individuals did you actually backtrack from other positives, so that they were true nodes of multiple contact points? So, one individual tests positive, “A,” I check their contacts.
But when you look at your entire matrix, how many crosspoints did you have between positives that focused on one individual? That is “A” contacted this person and that person so that there were multiple chains linking back to an individual rather than one individual and their contacts.
Ramanan Laxminarayan
Okay, so that’s a great question. Keep in mind that this is different from HIV for the simple reason that all the contact tracing was forward contact tracing, not backward contact tracing, because backward contact tracing doesn’t help you a lot with testing them [on] RT-PCR, right? You’re looking for people who are likely to be infectious, so as a public health measure, this is not a research study, this is a public health measure, we catch one person, then say how many people are you likely to have given the disease to?
I’m mulling on what Madhav had said. I think, Madhav, what you said makes sense with backward contact tracing, when you say, let’s say I had HIV, then the backward contact tracing makes sense to say, who might have infected me because that is another person with HIV. They were not doing that here because that backward tracing quickly becomes unproductive because you can’t pick up their positivity with RT-PCR and these states were not doing antibody testing.
Madhav Marathe
Yeah, let me think about it. I think that Aravind is also online and Anil is online too, [maybe] they have thought about this as well, but this aspect that, if you have a heterogeneous network, then you’re likely to reach in a biased way nodes with a high degree in the network. That doesn’t mean that they are necessarily a high degree in the transmission tree, basically. But perhaps, that’s the case too that you see, and I think that would be very interesting to study. I don’t know whether others have thoughts. I just thought about real-time, it’s really intriguing.
Aravind Srinivasan
Some of our experimental work did show that vulnerability is highly associated with degree, so that’s an interesting point.
Ramanan Laxminarayan
And this is for COVID?
Aravind Srinivasan
More generally, we didn’t do COVID-specific experiments. Yeah.
Ramanan Laxminarayan
Yeah, I’m just curious about, see, again, there’s a timing to this positivity, right? You have got to catch people in that first window of infection, so that’s really important. It’s not an on-off switch that stays on forever, you’ve got a period of time to catch that switch being on. I wonder if it works the same way for diseases like HIV as it does for COVID.
So maybe if you ran your model with that idea, that people have a period of infection after, would you still have the same amount of bias. I understand what you’re saying in a generic sense of, yes, you’re going to pick up those nodes because you’ll catch them because they’re connected to so many different people, so everyone will say, oh, I was next to that guy over there at the bus stop, and then very quickly, you find the guy at the bus stop and then you find more people.
Aravind Srinivasan
It’s the temporal aspect that should be modeled carefully, I see.
Ramanan Laxminarayan
Exactly.
Anil
Just one follow-up on that, so even in a temporal setting, this high degree node would have lots of neighbors, and some of them would get caught. Some of them might not because they missed the window.
Ramanan Laxminarayan
Right.
Anil
Let’s take this offline but I think it’s really important findings you have, Ramanan.
Golda Barrow
We have time for one more question. We have one from Abhishek, you mentioned that all contacts were tested, were they also asked to isolate at home, irrespective of symptoms, for some duration?
Ramanan Laxminarayan
Yeah, you had to do that. In the initial period, it wasn’t even at home, you had to go into a government facility and isolate. Then they changed the rules [so] that you could isolate at home. So for a long time, until about late July, early August, you had to go into the government’s quarantine facility.
Cool, for anyone on the call, do we have a similar dataset here in the US? I don’t know, from any state that has done this, from California or anywhere else?
Madhav Marathe
We are in touch with our state, Ramanan. Of course, I’m not just amazed by the analysis and the data but your ability to pull the data out and put it out there now.
Our state is collecting, like other states, and maybe once your paper is published, we’ll go back to the state of Virginia’s Department of Health and say that they could release data like this, in this form, because they are doing a fairly good job in terms of contact tracing as well.
We are of course strapped because of the number of folks who can do it, but we might want to study a similar issue in our state here. We might have a chance because we work with them very closely.
Ramanan Laxminarayan
Maybe you want to import some contact tracing experts from Tamil Nadu.
Madhav Marathe
That would be useful. We also have a small grant, Ramanan, from Google now, where we are supposed to study contact tracing, so we can talk to you in some more detail later.
Ramanan Laxminarayan
Sounds good. We have a lot of data now that we want to expand this work and do more with it, but the states have been very compliant and we hope to have continued cooperation.
Jim Koopman
You will get nothing like that from Florida, I assure you.
Ramanan Laxminarayan
Why? Don’t they want to know what’s going on?
Jim Koopman
Contact tracing is at a very low level and you can’t get any data from it at all.
Ramanan Laxminarayan
Interesting. I guess contact tracing is just very human-intensive. You need a lot of people. You need tens of thousands of people to do it.
Kathleen Alexander
I also just wonder, how much of it is actually whether or not you have a public health history of really large-scale contact tracing as just a way you do business? So when you have TB and HIV, it becomes such a part of your health infrastructure. I think that in countries that just don’t have that, they haven’t really been able to push it out again, the way that other countries in Africa or India do, where it’s just part of the fabric of public health.
Ramanan Laxminarayan
You’re right, you’re right. You’re absolutely right. I think HIV has helped.
Madhav Marathe
And Jim, didn’t you write a big polio work study in India? I mean, I know you played a very big important role [in polio eradication].
James Koopman
No actually, the polio [work] I didn’t do in India. But that last comment about the public health structure, I mean, across this country, HIV and TB have contact tracing in state health departments. It certainly didn’t help to get anything going here in Florida with regard to COVID.
Madhav Marathe
Let’s let Ramanan go, I think he has a class. Excellent talk, Ramanan. Thanks for sharing it before it appeared. Hopefully this will spark many more discussions and welcome to new folks who joined for your talk today, Jim being one, we had Sebastian Funk on the line for a while. Hopefully we’ll get more people [to] join us on this call. You’re welcome to share this link. Golda has done an amazing job, so we have many, many slots. We don’t have a fear of overrunning the slots on Zoom right now, so please do feel free to invite or share this link with others.
Thanks Ramanan.
Ramanan Laxminarayan
Thank you, thanks everyone. Bye bye.